spaCy
Last updated
Jun 17, 2023
# Introduction
- deep-learning-based NLP system
- learn and summerize the syntax model from Corpus,
# 安装
1
2
3
4
|
// 安装
pip install spacy
// 下载模块
python -m spacy download en_core_web_sm
|
# 基本用法
1
2
3
4
5
6
7
8
9
10
11
12
13
14
|
# 1. load model
import spacy
nlp = spacy.load("en_core_web_sm") # lang
# 2. parse
# Doc 对象是 spaCy 里的基础 statement 对象
analyzed = nlp("I shot an elephant in my pajamas.") # Doc object
# 3. Visualizing
spacy.displacy.render(analyzed, options={'distance':100})
# 4. explain 某个标签
print(spacy.explain('poss'))
# possession modifier
|
1
2
3
|
# 打印进阶信息
for token in doc:
print(token.text, token.lemma_, token.pos_, token.tag_, token.dep_, token.shape_, token.is_alpha, token.is_stop, sep='\t')
|
Dies |
dieser |
PRON |
PDS |
sb |
Xxxx |
True |
True |
ist |
sein |
AUX |
VAFIN |
ROOT |
xxx |
True |
True |
mein |
mein |
DET |
PPOSAT |
nk |
xxxx |
True |
True |
erster |
erster |
ADJ |
ADJA |
nk |
xxxx |
True |
True |
Satz |
Satz |
NOUN |
NN |
pd |
Xxxx |
True |
False |
. |
– |
PUNCT |
$. |
punct |
. |
False |
False |
# 可视化
# displacy
1
2
|
from spacy import displacy
displacy.render(doc)
|
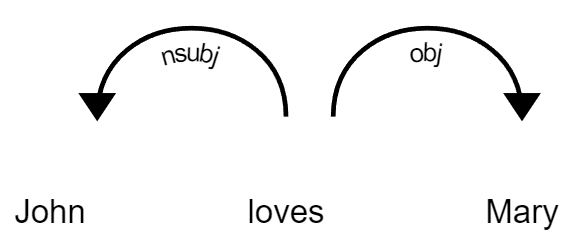
# 注意
BEACHTE: unterschiedliches PP-Attachment englisches vs. deutsches Modell!
en
: PP als Dependent vom Kopf der VP (shot)
de
: PP als Dependent vom Kopf der NP (Elefanten)
Grund: strukturelle Ambiguität! (Modelle favorisieren jeweils andere Attachment-Art)